Claim Management
Why Data Analytics is the Most Powerful Tool in Your Claims Management Software
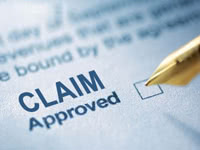
Following in the footsteps of CRM, ERP, and others that have made the traditional methods of organizing their respective data sets obsolete, claim management software aims to digitize and automate as much of the insurance claims process as possible to save time tracking down claimant information and save money by providing models for processing future claims. Heavy reliance on data and analytics-based decision making are nothing new to claims management, but the explosion of big data has precipitated a resurgence of focus on the potential for analytics to bring companies ever-closer to perfect operational efficiency.
When considering the role that analytics should play in informing decision making, companies must first identify those claims which account for the majority of loss. This can be accomplished using analytics software, or by traditional methods of data comparison to determine the best and worst-performing claim groups and trace them back to the processes that first accepted the claims. For many agencies, evaluating even a moderate number of claims can quickly become a task that exceeds the capacity of their risk managers whose time is better spent reviewing outliers and interacting with clients than individually viewing every claim. To remedy this, analytics within claims management software develop forecasts called predictive models. As the team behind Analytics Plus, a claims management analytics provider, stated in a recent whitepaper:
In many claims management organizations a small percent of claims incur a large percent of total payments made to claimants. Predictive models can be created to identify this small percent of cases early in their development for faster intervention.
More specifically, the power of predictive modeling is in its ability to “learn” as much as it is in its ability to quickly generate reports. Learning in the context of predictive modeling refers to the recognition of statistically relevant (and conversely, potentially misleading) data within large sets. This allows companies to adjust models to appropriately match the reliability of data within a given field. To provide a use case, Analytics Plus uses medical claims to explain the concept of learning as it pertains to predictive modeling:
For example, if a model was built from health care data which had very few cases of MRI and CAT scans historically, it most likely will underestimate the current cost of claims. The higher weighting of recent data and incorporation of new data post deployment cannot be overlooked.
Because a small number of claims typically account for the majority of cost to the insurer, taking information such as the number of previous claims into account when creating predictive models is paramount to a particular model’s success since, “early intervention of a potentially expensive claim is the goal of claims management.”
Not only do advanced predictive models help improve the efficiency of claim acceptance, but have immense value when applied to the evaluation of fraud. In the past, fraudulent claims were only discovered when they stood out as clear anomalies and even then the potential for human error meant that a certain number of claims would fall through the cracks. No longer says Pat Speer of Insurance Networking News who notes that:
…fraud discovery no longer hinges on when the fraudster is most human (makes a mistake and gets caught); instead, it gives equal play to data that can be used to back a proactive strategy to predict and prevent as well as to prove its case.
It is clear that, “while individual variables can be used to predict the potential loss, the real power of predictive modeling comes from combining numerous variables to identify patterns that couldn’t be recognized without the aid of these statistical tools,” but how do companies act on the data that predictive modeling provides? As Deloitte suggests in a 2011 whitepaper:
Claims placed in the worst segment can be assigned to the most experienced personnel and given the appropriate attention and oversight required. The best claims can be routed to a service center or entry-level worker, decreasing operational costs and freeing up resources for the most complicated claims.
By shifting the focus of senior agents to claims that have the highest potential for loss, unnecessary costs can be mitigated while background analytics use outlying data to reformulate predictive models and avoid acceptance of such claims in the future. The intrinsic value in implementing an automated solution should be reason enough to consider migrating to claims management software if your company does not already use one, but the value of the analytics behind today’s claims management software extends far beyond simple time saving to benchmarking, performance measurement, and iterative model generation.
Want to read more insider-perspective posts? Browse our entire archive of tips and advice from software industry experts by visiting the Industry Insights section of the Business-Software.com blog.